Subscribe
According to the OECD, “Unstructured data are by far the most frequent type of data and thus provide the greatest potential for data analytics today.”
You come across this type of data daily without even realizing it. For instance, the barrage of customer support tickets with text-based information is unstructured data. Because of their lack of uniformity, you can't analyze them in bulk without the help of advanced analytics tools.
To help you navigate this, we’ll share an overview of unstructured data analytics and how it can help organizations like yours gain actionable insights that significantly impact your bottom line.
We'll also discuss why it's important for customer experience (CX) teams, explore which data types are most often analyzed, and show you how unstructured data analysis works.
What is unstructured data?
Unstructured data is a form of data that doesn't have a predefined structure or format, making it difficult to manage and organize it. This data type is often found in business documents, such as SMS data, call transcripts, chatbot messages, emails, PDF documents, and more.
For example, it’s hard to categorize your social media posts into definitive categories since each post has a different structure, topic, and goal. The lack of uniformity is what makes it unstructured.
At Dimension Labs, we also refer to it as “dark data” because of its untapped potential. As dark data tends to be more information-dense due to its nature, analyzing it can open a doorway into unseen yet actionable insights for businesses.
Structured data vs. unstructured data: what’s the difference?
Before we explore how to analyze unstructured data, let's explore the key differences between structured and unstructured data. It should help you understand why analyzing unstructured data is not as simple as it seems.
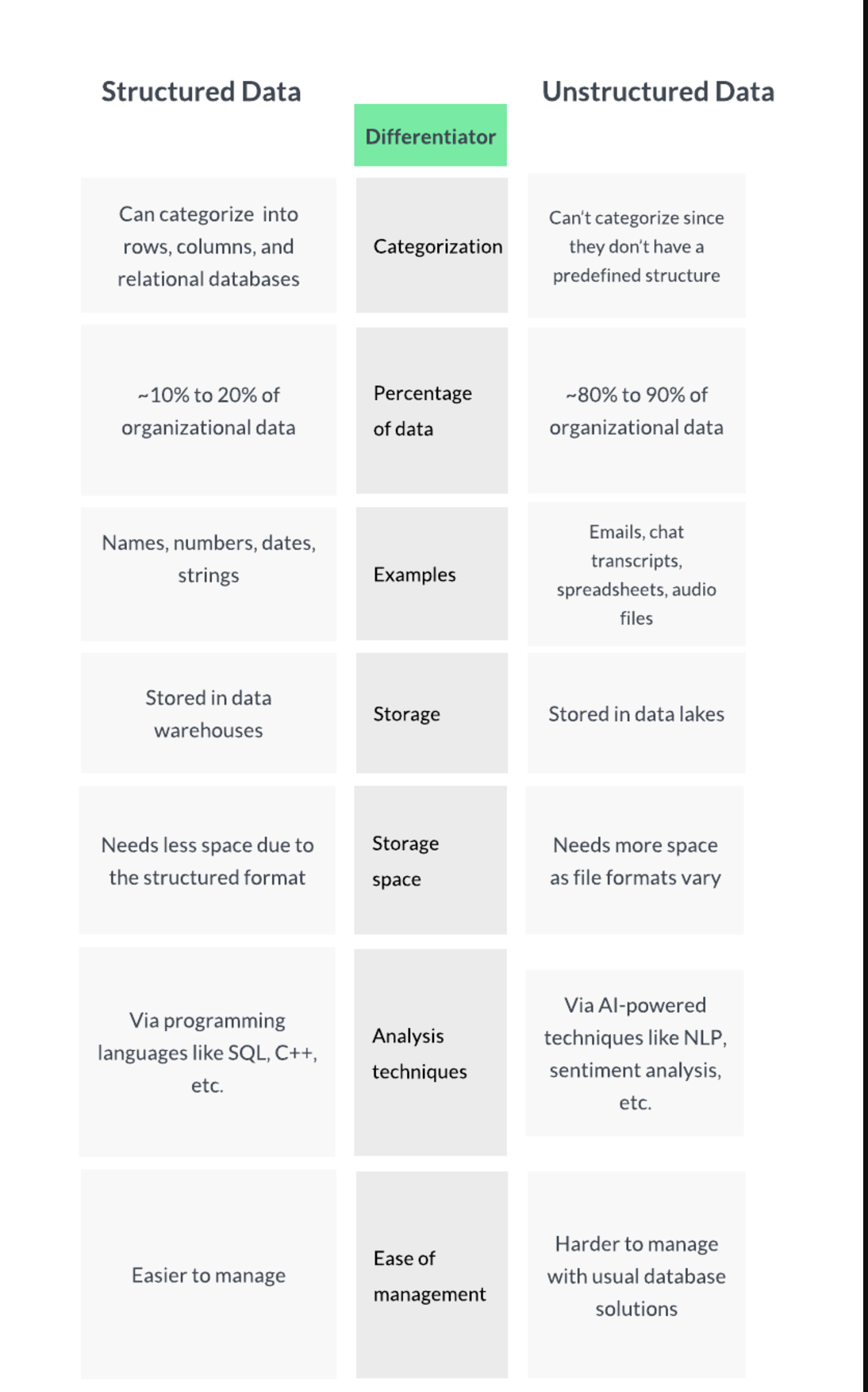
Examples of unstructured data
Here are a few examples of unstructured data you can generate in a CX environment:
- Customer feedback: These can be sourced through third-party review sites, chatbot transcripts, email feedback, customer feedback forms, blog comments, etc.
- Open-ended surveys: You can get this from open-ended responses you receive from market research, customer feedback, event registration forms, post-service feedback forms, etc.
- Emails: You can source this from any email you receive from customers. It can include unprompted customer feedback, customer support requests, responses to regular email communication, and more.
- Chatbots: You can source this from your chatbots which can be present on any channel. For instance, it's common for companies to use multiple use cases or page-specific chatbots on their website, help center bots, social media bots, and internal support bots. The data can be in the form of text transcripts or audio files (voice calls with customers).
- Social media: You can source this through the channels that your company is active on. For example, LinkedIn, Twitter, Reddit, Facebook, Instagram, Discord, etc. The files can be in the form of text or image/video format, depending on what the request is.
- Websites: This can come from blog comments, contact form data (open-ended), product reviews, frequently asked questions, and more.
- Customer support calls: These don't necessarily have to be handled by a bot. If you run a dedicated help center, exchanges between the customer and support representative act as a good data source for CX teams.
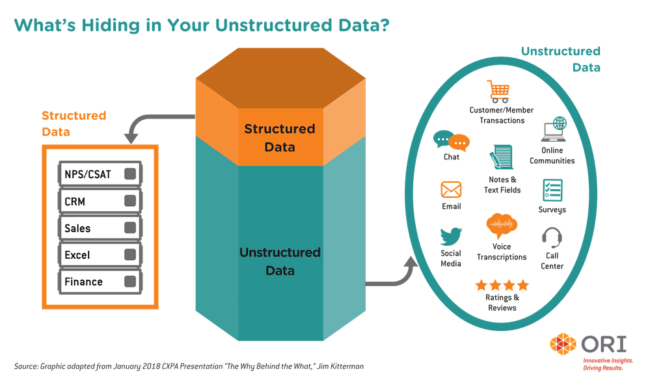
How to analyze unstructured data
Analyzing unstructured data is crucial as it can yield valuable insights into customer behavior and trends. It typically involves three steps:
- Collecting relevant data from various sources like email, chatbots, social media, etc.
- Cleaning and preprocessing the data using data analysis tools
- Applying multiple AI-powered data analysis methods like sentiment analysis to extract insights
Data collection usually starts with gathering data from unstructured data sources. Depending on the context, this could include social media posts, customer reviews, market research surveys, news articles, etc.
Once you have relevant data, clean and preprocess it to ensure that only valid and useful information is used for analysis. It usually involves removing any irrelevant or invalid entries from the dataset and formatting the remaining entries into a suitable format for analysis. For example, text data like "Hi, how are you?" are removed to include only contextual data.
Once the dataset is ready, it can be analyzed using various methods such as text mining, sentiment analysis, or machine learning. Here's how they help:
- Text mining: Identifies patterns and trends in textual sources by searching for keywords or phrases within documents.
- Sentiment analysis: Identifies emotional sentiment towards a specific topic in text-based sources such as social media posts or customer reviews.
- Machine learning: Identifies patterns and finds correlations between different variables, which can provide valuable insights into customer behaviors and preferences.
You should choose the technique based on the type of data you have. For instance, text mining would be a good fit if you need to analyze 1000 customer support tickets. On the other hand, if you're analyzing voice call transcripts, you will need advanced conversational AI tooling.
Ultimately, this process requires specialized knowledge and technical expertise to achieve accurate results. If you’re curious about how to unlock your dark data, check out our recent webinar on the topic below:
Use cases for unstructured data analytics
Here are a few use cases of unstructured data analytics tools like Dimension Labs—especially for customer support professionals:
Show customer data by channel
With the help of these tools, users can dive deep into relevant metrics like customer sentiment and resolution rate across SMS, email, voice call, and more. Businesses can then use these insights to verify if their support systems, like chatbots, are working optimally or need adjustment for better results.
Highlight negative sentiment conversations
Using unstructured data, customers can identify negative reactions toward different types of support requests, giving them insight into what issues are causing the most dissatisfaction. If there's a noticeable increase in customers reporting specific issues like password change, Dimension Labs will highlight it and alert you. It helps you tackle the problem before it gets out of hand.
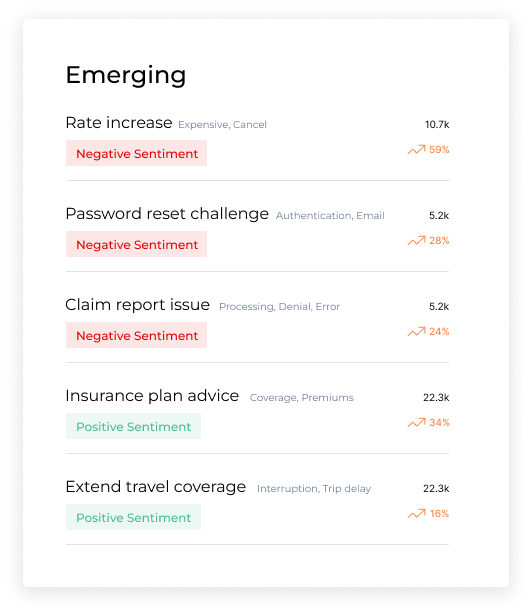
Determine the top customer support requests
Dimension Labs can help you identify trends like customer channel preference for specific use cases. Based on that, it can detect which topics and channels have the highest frustration, allowing you to better coach agents or redirect customers accordingly.
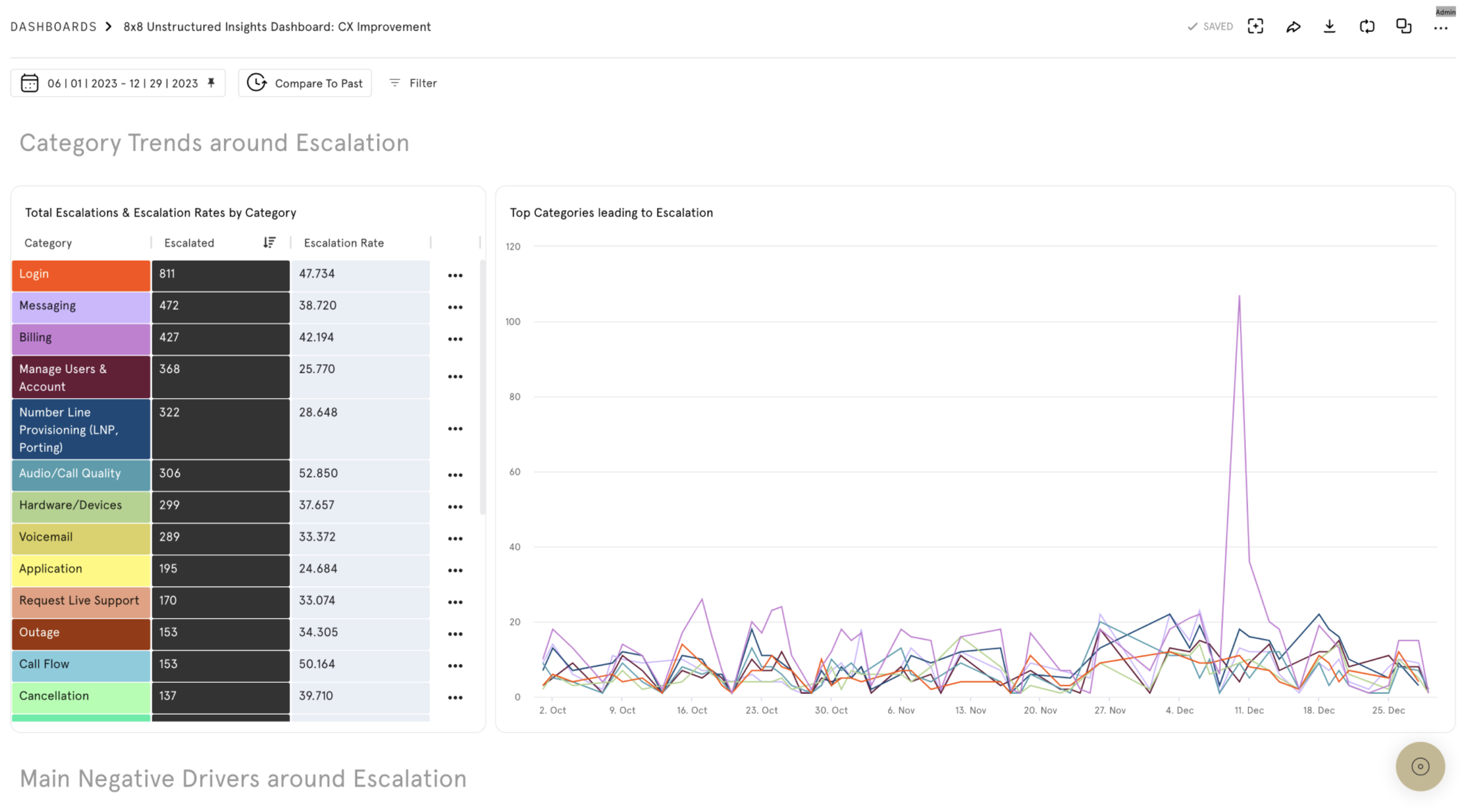
Analyze tone and sentiment for agents to improve or train them
You can quickly analyze tone and sentiment to understand the customer's experience. This capability helps you access Key Activities categorized by topics to act on them. For example, you can see what percentage of customers seek information or make transactions. It combines this data with context like resolution rate, average time spent, and sentiment so you can create a data-driven strategy to train your support agents.
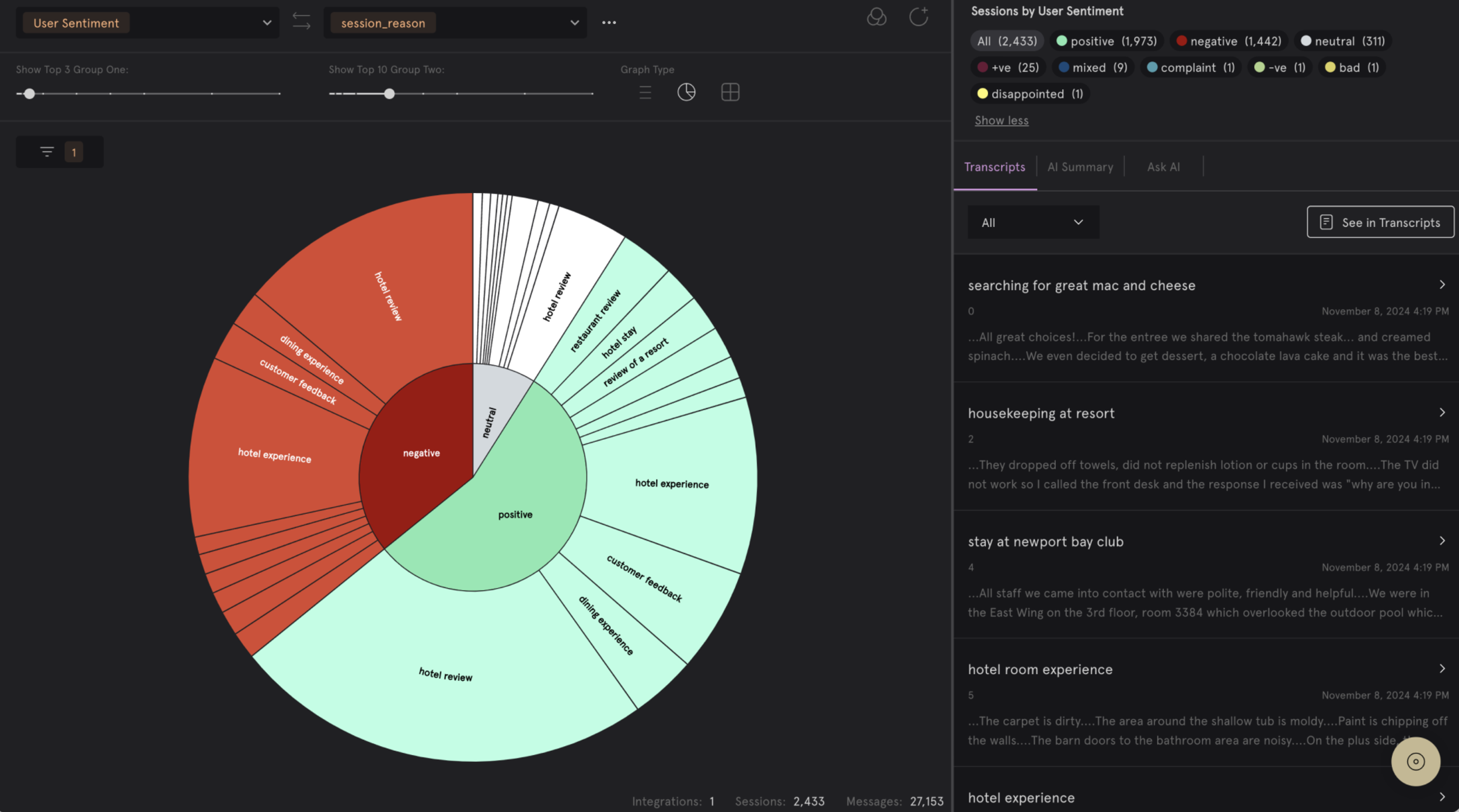
Show new trending support topics
With its advanced unstructured data search and extraction capabilities, Dimension Labs can list topics emerging in customer conversations. It allows your internal teams to proactively prepare for a surge of user inquiries, helping ensure that customers have satisfactory experiences.
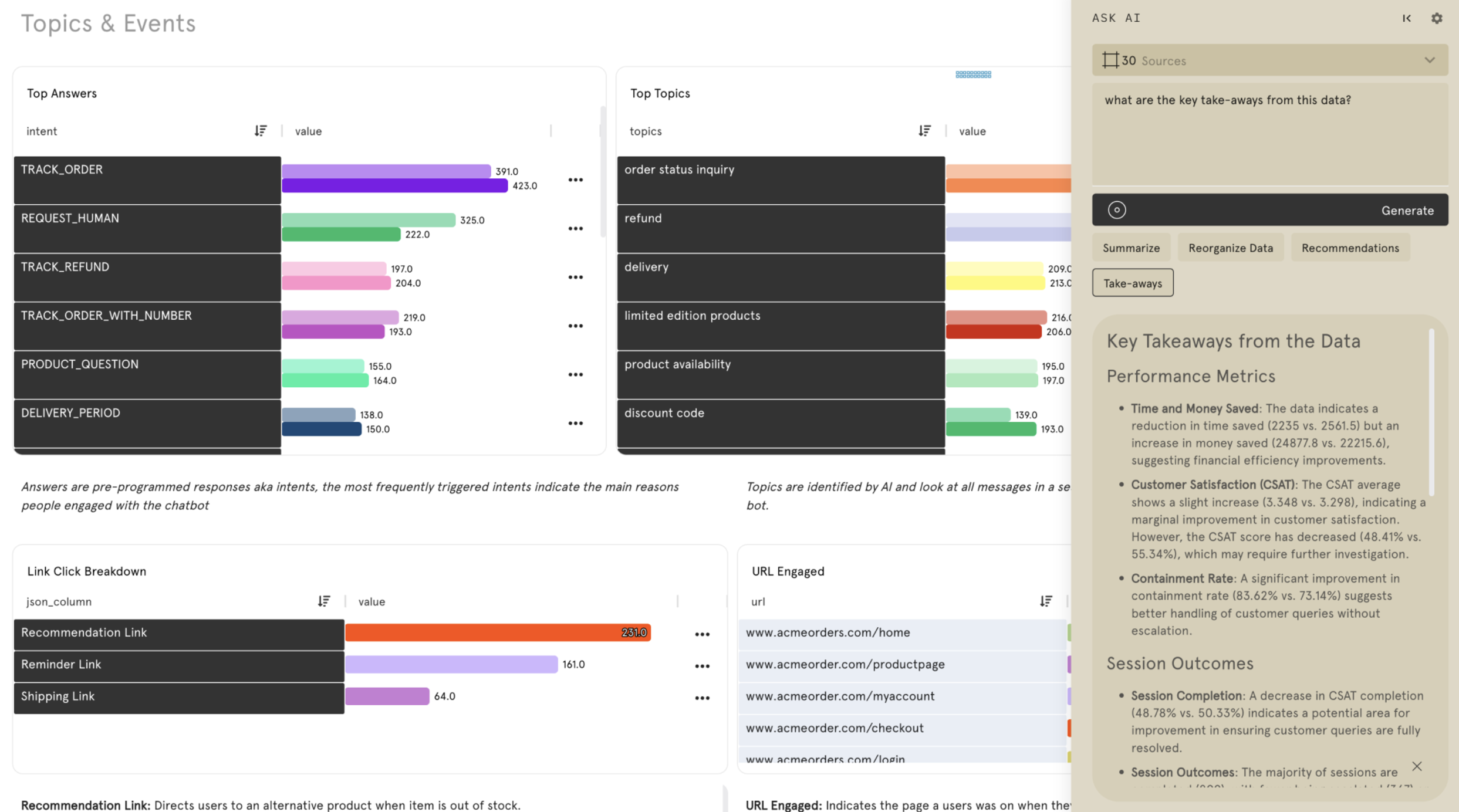
Analyze your unstructured data using Dimension Labs
Unstructured data is a potent tool for customer support teams, providing valuable insights and advantages you can't find elsewhere. This data type provides a more comprehensive view of customers' needs and experiences than structured data alone, leading to more informed decisions and better customer satisfaction.
In addition, you can stay ahead of the curve by quickly identifying trends or issues that may arise. Tools like Dimension Labs allow customers to customize their experience when interacting with a business or organization. These unique benefits clarify why customer support teams should use unstructured data to maximize efficiency and effectiveness.
FAQs for unstructured data analytics
- What is unstructured data?
Unstructured data is any type of data that does not have a predefined structure or format. It could include audio, images, videos, email messages, and web pages. It requires specialized tools such as NLP or image processing algorithms to extract information from it. Unlike structured data, which is organized in a tabular form and can easily be queried using SQL, unstructured data needs to be heavily processed to extract valuable insights.
- What tools are used for analyzing unstructured data?
Any tool that offers the capabilities of NLP, text mining, or machine learning can help you analyze unstructured data. Dimension Labs is an excellent example for this purpose. You can process and analyze your customer support data through our NLP model to determine how your customer truly feels about your product/service.
- Why is unstructured data difficult to analyze?
Unstructured data lacks structure and does not adhere to a predefined data model. This lack of structure makes retrieving and interpreting information from this type of data more challenging. The data can be messy and incomplete, making it hard to parse necessary details without AI-powered data analysis tools.